Motivation: According to the statistics provided by Centers for Disease Control (CDC), 795,000 people in the United States suffer from the effects of stroke every year. Of all the patients diagnosed with a stroke, 88% of patients experience hemiparesis, or partial paralysis, of the upper limb. However, diagnosis of any grasp impairments is heavily dependent on the visual inspection of physicians and therapists and relating questionnaires, as proposed by Barker et al. Thus, we present an objective, quantitative measurement of grasp performances in this work, where we utilize a sensorized glove system that measures grasp poses and contact forces simultaneously.
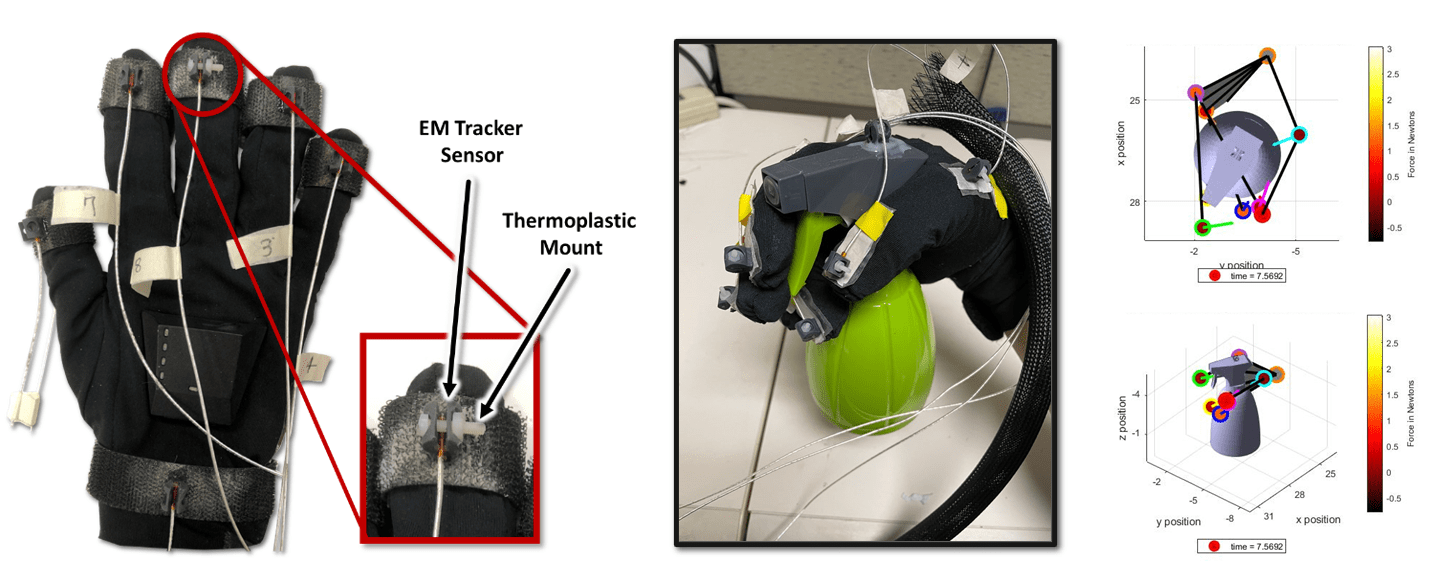
Research Focus: To characterize grasp pose and contact dynamics simultaneously, we have used a pre-made tactile pressure sending glove, the TactileGlove by Pressure Profile Systems, and modified it such that a set of electromagnetic trackers can be mounted (Figure 1). Grasp pose is characterized by tracking the Cartesian coordinates of the fingertips, the wrist, and the base of the thumb using a set of electromagnetic trackers powered with the TrakStar 3D Guidance system by Ascension Technology. The contact pressure distribution at the palmar side of the hand is measured using the TactileGlove, embedded with 52 capacitive pressure sensors in the nylon fabric glove. The glove is capable of measuring force values between 0.04N and 70N at a rate of 40 Hz.
Using the grasp characterization glove, a human subject study was performed on nine subjects where each subject was to grasp 10 random objects from a pool of 36. To visualize the grasp dataset from the human subject study for a better understanding of pose and contact dynamics, the grasps were represented using animations that show the grasp poses and contact forces (Figure 2). The grasp pose was displayed using a skeletal representation of the hand where critical contact points of the hand are represented with circles with different outline colors. The contact forces were visualized with color infills and arrows with their length proportional to the contact force magnitude.
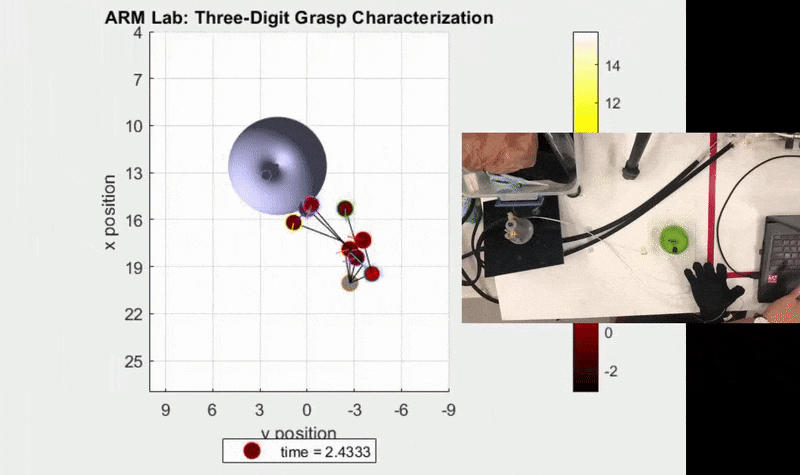
Findings and Implications: Using the grasp kinematic and dynamic data, we have performed principal component analysis and linear discriminant analysis to evaluate the usefulness and efficiency of adding force features to the grasp kinematics. Our system was able to predict object types based on grasp pose and contact forces with a mean of 76.6% accuracy as opposed to 70.5% accuracy from estimation using only postural information. The result is comparable to related works, ranging from 40% to 83.5% depending on the usage of a convolutional neural network.
With this system, physicians can utilize the characterization glove to develop a quantitative kinematic and kinetic modeling of the patient’s hand compared to the traditional methods to design a personalized therapeutic program for each patient. More applications of this system include facilitating grasp analyses for sports therapy, creating simulations of postural synergies for the design optimization of manual tools, and creating an empirical basis for the design of patient-specific powered robotic prostheses.
People
- Joshua Lee (PhD Student)
- Derek Mu (Masters Student)
Publications
- J. Lee, D. Mu, and F. Hammond III, Characterization of Grasp Performance Using a Sensorized Glove System, Frontiers in Biomedical Devices, 2021